Deep Learning Infers Structures of General Lyotropic Phases from Small Angle Neutron Scattering
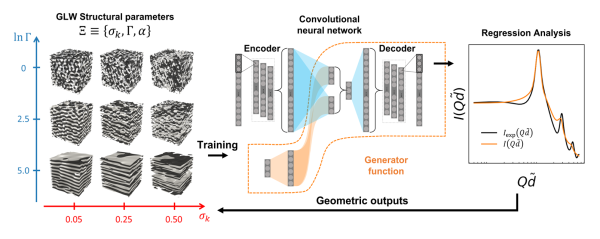
Scientific Achievement
Outlined a novel strategy that integrates convolutional neural networks and stochastically regulated matter wave field into a regression analysis framework for inferring real-space structures of general lyotropic phases.
Significance and Impact
Deep learning surpasses traditional modeling for complex lyotropic phases, including fused topological features. Also emphasizes effectiveness in addressing challenges in soft matter structural analysis and beyond.
Research Details
- This approach's feasibility was tested through computational benchmarks and small angle scattering demonstrations.
Chi-Huan Tung, Yu-Jung Hsiao, Hsin-Lung Chen, Guan-Rong Huang, Lionel Porcar, Ming-Ching Chang, Jan-Michael Carrillo, Yangyang Wang, Bobby Sumpter, Yuya Shinohara, Jon Taylor, Changwoo Do, and Wei-Ren Chen,
“Unveiling mesoscopic structures in distorted lamellar phases through deep learning-based small angle neutron scattering analysis”
Journal of Colloid and Interface Science, (2023). DOI: https://doi.org/10.1016/j.jcis.2024.01.003