Virtual Node Graph Neural Network for Full Phonon Prediction
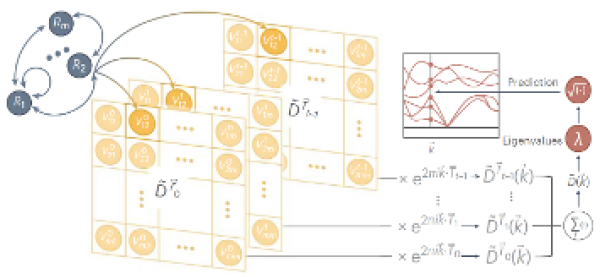
Scientific Achievement
Virtual node graph neural networks (VGNNs) are proposed to make predictions of properties with material-dependent dimension, using the atomic coordinates as the only input. Γ-phonons and full phonon dispersion are predicted from the crystal structure to demonstrate the capability and potential of VGNNs.
Significance and Impact
The rapid prediction of phonon dispersion has significant implications on neutron spectroscopy. The flexibility offered by the VGNNs opens the door to direct predictions of a broad range of materials properties from the structure.
Research Details
- Three virtual node approaches are developed to predict phonons.
- The VGNN was used to generate databases of Γ-phonons for over 146,000 materials and phonon band structures for zeolites.
"Virtual node graph neural network for full phonon prediction," Nature Computational Science 4, 522-531 (2024).
DOI: https://doi.org/10.1038/s43588-024-00661-0